Machine Learning vs Deep Learning
- Goutham Ravikumar
- Jul 7, 2020
- 4 min read
When we see and understand the latest advancements in Artificial Intelligence (AI), it seems to be overwhelming, but if you’re looking to learn the basics as per your choice, then you can narrow many such AI innovations down to two concepts, i.e., machine learning and deep learning. These terms often seem like they're interchangeable buzzwords, and that is why it's important to know the differences between the two.
And these differences are something that everyone should know, and examples for machine learning and deep learning can be found everywhere. For instance, have you ever wondered as to how would Netflix know which show you’ll want to watch next, or how Facebook analyses whose face is in a photo, or what is it that makes self-driving cars a reality, and how will a customer service representative know that you will be really satisfied with their support even before you take a customer satisfaction survey or feedback?
So now, what are these concepts that are dominating the conversations about the two terms and how exactly are they different from each other?
Deep learning vs machine learning
The easiest takeaway for understanding the complete difference between machine learning and deep learning is to know that deep learning is a part of machine learning. In other words, and to be more specific, deep learning is considered to be an evolution of machine learning. It uses a programmable neural network that will enable machines to take proper and accurate decisions without actually taking any help from humans.
Next, before we move into the topic, we should first know the meaning of Machine Learning for better understanding. Hence, we will look into that and then jump into the topic.
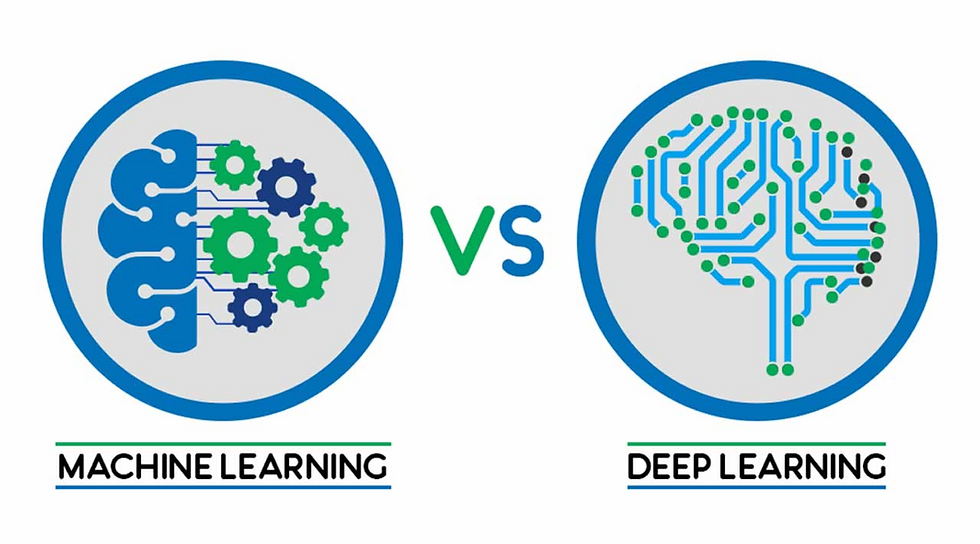
What is this Machine Learning?
Machine learning is one of the applications of Artificial Intelligence (AI) which provides systems the ability to automatically learn and improve from its experience without being programmed. Machine learning mainly focuses on the development of computer programs that can access data and use it to learn for themselves.
A simple example of a machine learning algorithm is an on-demand music streaming service. For them to make decisions on which new songs or artists to recommend to the listener, machine learning uses its algorithms and suggests songs based on the listener’s preferences by comparing it with other listeners who have a similar musical taste. This technique, which is often simply touted as AI, is used in many services that offer automated recommendations.
Machine learning solves all sorts of automated tasks that come across multiple industries, right from the data security firms that disturb the malware functioning to the finance professionals who want alerts for trades that are favorable to them. The AI algorithms are programmed to constantly be learning in a way that simulates as a virtual personal assistant and this is something that they do quite well.
Difference between Deep Learning and Machine Learning
Practically, deep learning is just a subset of machine learning. In fact, deep learning technology is machine learning and functions in a similar way. However, its capabilities are different.
Though the basic machine learning models can become better at whatever their function is, they still need some guidance. If an AI algorithm starts giving an inaccurate prediction, then it’s obvious that the engineer has to step in and make adjustments to it so that it would perform better. When it comes to deep learning models, the algorithm will look after and determine if a prediction is accurate or not through its own neural network and that too on its own.
How does deep learning work?
Deep learning models are designed to continually analyze data with a logic structured similar to how a human would draw conclusions. To achieve this, deep learning applications use something called a layered structure of algorithms called an artificial neural network. The design of this neural network is inspired by the biological neural network of the human brain, and this leads to a process of learning it is far more capable than of all the other standard machine learning models.
It’s a bit tricky to say that a deep learning model doesn’t draw incorrect conclusions at all. Just like other examples of AI, deep learning too requires lots of training to get the learning processes correct and for it to get going. But when it works as it’s intended to, functional deep learning is often considered as a scientific marvel which many think as being the backbone of true artificial intelligence.
One of the great examples of deep learning is Google’s AlphaGo. Google had created a computer programming by using its own neural network that learned to play the abstract board game called Go, which is known for requiring sharp intellect and intuition. By playing against professional Go players, AlphaGo’s deep learning model learned how to play at a level never seen before in artificial intelligence. It caused quite a stir when AlphaGo defeated multiple world-renowned “masters” of the game. It not only could grasp the complex techniques and abstract aspects of the game, but it was also becoming one of the greatest players of the game as well.
Conclusion:
In the coming future, there is no doubt that deep learning is going to be everywhere and people should not really wonder looking at the advancements that Machine Learning and Deep learning are going to make in the future. Because Deep Learning has its own capabilities of learning and understanding things, it is important to keep in mind that algorithms, methods, and approaches will continue to change and definitely advance in the near future.
If you are looking to start a course with AI & ML, we at Infimind Institute, help achieve the dream and goal of by conducting an academic course of Artificial Intelligence and Machine Learning.
Comentarios